Build Your Own AI: Efficient Medical AI development using Active Learning
Artificial intelligence (AI) is revolutionizing clinical practice, yet developing and validating AI solutions remains a formidable challenge, especially for medical professionals. The need for technical programming expertise required to develop AIs, combined with the labor-intensive process of annotating data required for training render development of AI solutions incompatible with the demanding schedules of expert physicians.
Our work lowers these barriers and democratizes AI development for physicians. Leveraging the power of Active Learning (AL) and an innovative method for selecting high-value examples, our approach drastically reduces the volume of annotated data required. This not only eases the annotation burden but also significantly enhances the performance of medical image classification AIs.
Furthermore, our open-source pipeline includes comprehensive, user-friendly setup instructions that require no programming expertise. Rigorous evaluation in training AI for Gastro-Esophageal Junction assessment using the Hill classification demonstrated that our AL pipeline produces superior results compared to traditional methods. Notably, in this example, our AL-pipeline excelled in identifying under-represented grades (3 and 4), resulting in remarkable performance improvements with fewer annotated samples. This breakthrough underscores the transformative potential of our AL approach in advancing medical AI training and making AI development accessible to all physicians.
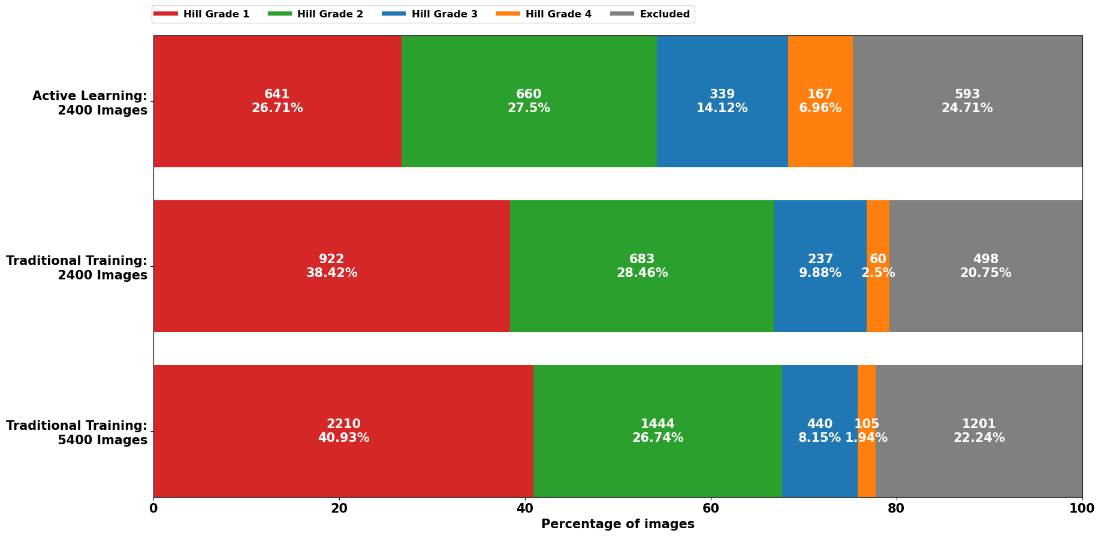
Publication
I. Kafetzis, K.H. Fuchs, P. Sodmann, J. Troya, W.G. Zoller, A. Meining, A. Hann, Efficient Artificial Intelligence-based Assessment of the Gastroesophageal Valve with Hill Classification through Active Learning, (2024), Scientific Reports, DOI: 10.1038/s41598-024-68866-x
Go to publication
Download software